Nest choice in turtle ants is influenced by spatial constraints
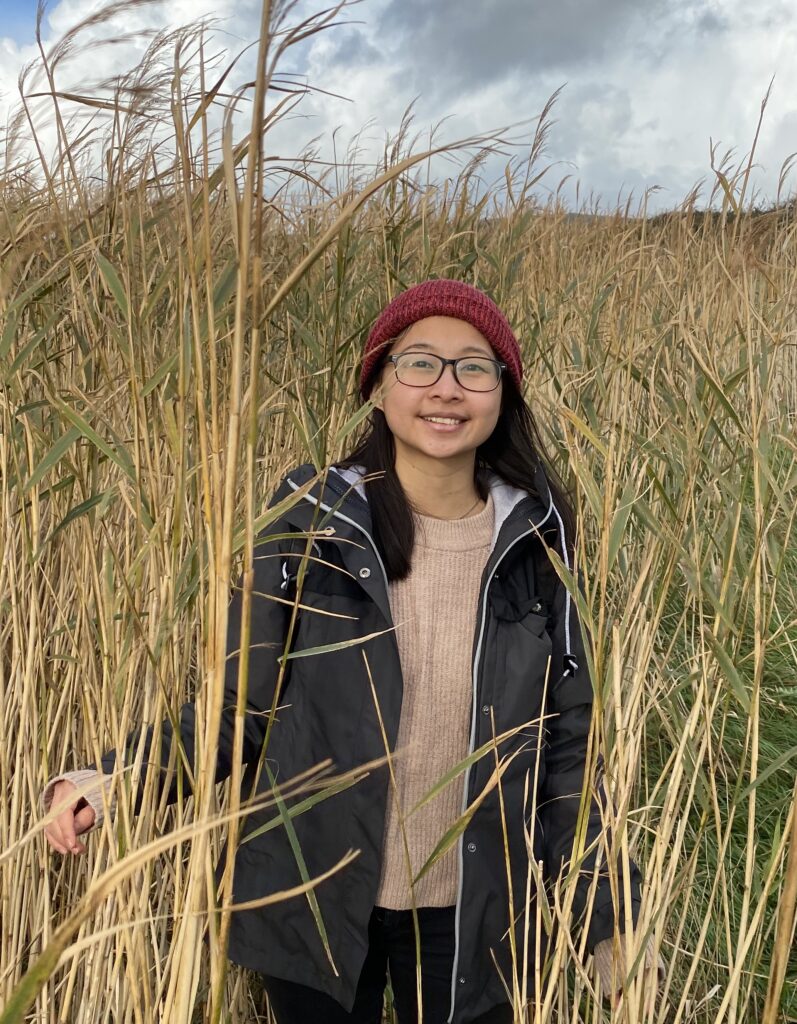
In the recent article “Nest choice in arboreal ants is an emergent consequence of network creation under spatial constraints” published in Swarm Intelligence, Joanna Chang, Scott Powell, Elva J.H. Robinson, and Matina C. Donaldson-Matasci investigated how arboreal turtle ants choose their nest sites when various sites and connections are available. They revealed that nest choice is influenced by spatial constraints. Here, first author Joanna Chang highlights the main points and shares some pictures.
An Interview compiled by Alice Laciny, Patrick Krapf, and Felix Oberhauser
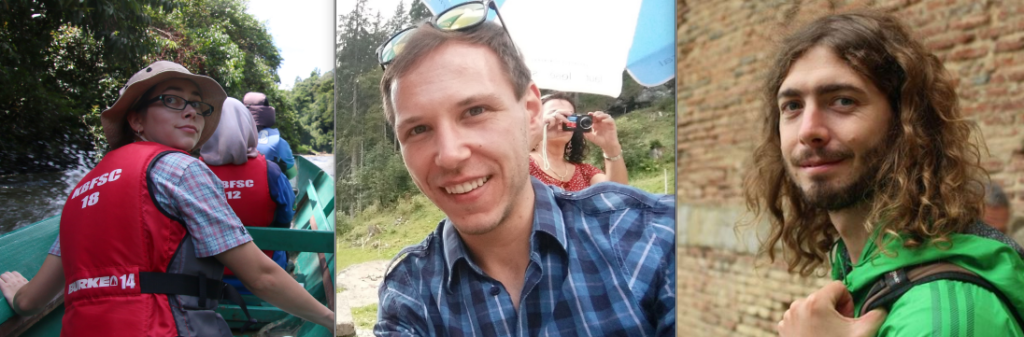
MNB: Could you tell us a bit about yourself?
JC: I am currently a PhD student at Imperial College London in the UK. Previously, I completed my Bachelor’s at Pomona College in the US with a major in biology and a minor in computer science. I’ve always been interested in nature and insects, but I fully discovered my love for social insects during my Bachelor’s. There, I was involved in studies on honeybees and turtle ants, and I realized how intricate and fascinating their social dynamics were. With a budding interest in computer science, I also saw the potential of using computational tools to study these complex systems. I have full control over the system in a computational model, so I can break down their behavior into simple rules and pinpoint the factors that explain the patterns we see in the laboratory experiments. I think this is a powerful approach, so I have been working at the intersection between biology and computer science ever since.
MNB: Could you briefly outline your recently published article in layman’s terms?
JC: Turtle ant colonies occupy multiple nests, and these nests form the nodes of networks that the ants use to travel and distribute resources between. We were curious about how turtle ants form these networks within spatially-constrained environments, and we studied this by looking at how they choose new cavities to occupy. In a laboratory setting, we presented the colonies with two sections: one with more connected pathways between the cavities and one with fewer. We studied this using two arenas with different spatial configurations, and we were surprised that there was a preference for the more connected section in one configuration, but not the other. Using a computational model, we were able to replicate the different behaviors in the two configurations, showing that their behavior can be explained by a few rules. First, ants move around rather randomly in the initial stages of exploration. Second, ants are more likely to aggregate at nests that already have other ants inside. When their random movement is constrained to the pathways in their environment, certain patterns of nest aggregation (and thus nest choice) consequently emerge. This means that nest choice can be largely driven by the spatial layout of the surrounding environment.
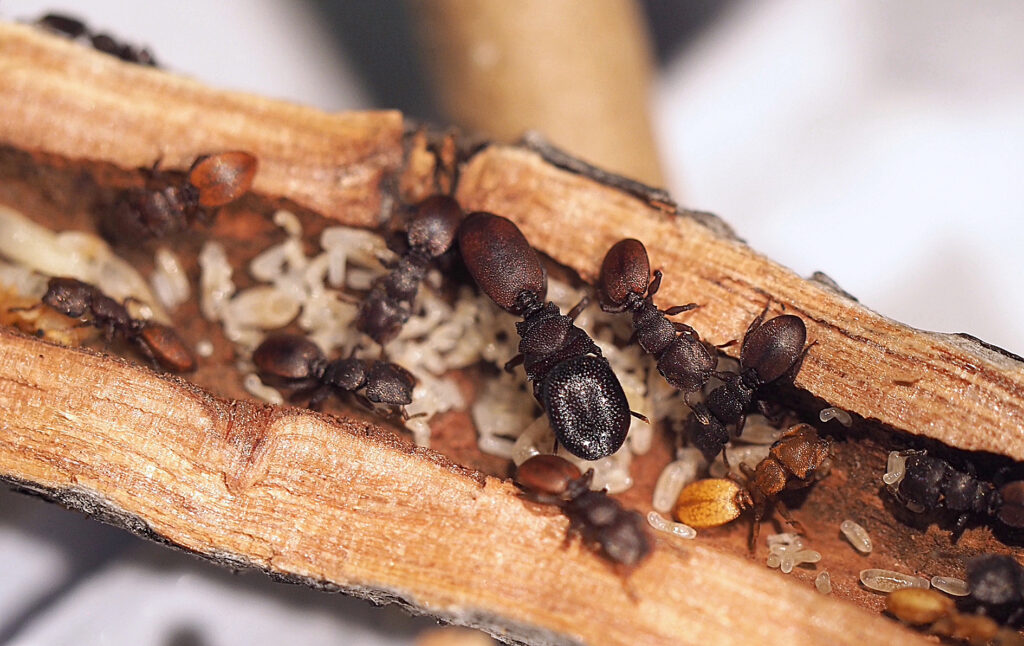
MNB: What is the take-home message of your work?
JC: A large takeaway from this study is that collective behavior should be examined in the context of the environment and its constraints. At the beginning of our study, we thought that the ants would choose nests based on a preference for certain nest network properties like connectivity and robustness. However, through our computational model (which did not model preference), we saw that their behavior could be explained by simple rules and how these rules interact in their particular environments, without any preference. For example, we saw that the number of junctions in the environment could affect the encounter rate of ants at each nest cavity, and this eventually determines the nests that they choose to include in their network.
Cavity for lab experiments (© Joanna Chang) Configuration (© Joanna Chang).
MNB: What was your motivation for this study?
JC: Many organisms rely on biological transport systems to distribute vital resources, and we were interested in how these systems are built and used. We were especially interested in studying the networks of turtle ants because they are an arboreal ant species. In contrast to their terrestrial counterparts, arboreal ants have more constraints on their networks since they are limited to the topology of the trees they inhabit. Turtle ants are polydomous – meaning that they occupy multiple nests – and they occupy pre-existing cavities instead of creating their own, so their nests are also limited resources. Also, turtle ant networks may face more disturbances since they are exposed to wind and other elements that can destroy their nests and pathways. As a result, we were interested in how turtle ant networks may be built differently to adapt to these additional constraints and disturbances.
MNB: What was the biggest obstacle you had to overcome in this project?
JC: I had always been interested in biology and had done my fair share of backyard experiments with insects since I was little, so I was comfortable (but still very eager) to do the laboratory part of the project. However, I had just started dipping my feet in computer science when I decided to embark on the modelling part of the project. It was difficult to condense all of the complexity and idiosyncrasies of a natural system into a computational model. It required a shift in perspective, and it meant that I had to strip the system down into the components that really mattered. This was also frustrating at times, because I wanted to add more and more components to the model to reflect the real system, but of course, that is not possible. In the end, I had to recognize that all models are imperfect, but many can still be useful. As I learned to approach the science from this perspective, it helped me see that there was a certain elegance in how the ants’ collective behavior can be driven by a few simple rules.
MNB: Do you have any tips for others who are interested in doing related research?
JC: Collaborate closely with both field biologists and modelers. For this project, I studied turtle ant behavior in a laboratory setting and then used a computational model to understand our findings. I was lucky to collaborate with Scott Powell, who is experienced in both field and lab research, and Elva Robinson, who is experienced with modeling ant behavior. My advisor, Matina Donaldson-Matasci, has also worked on both sides. I relied on their expertise to connect the two parts of the project together. Staying close to the field data made sure that the model remained true to the biology. Meanwhile, expertise about the modelling process helped me capture the essence of the system with the right levels of abstraction.
MNB: Where do you see the future for this particular field of ant research?
JC: I am excited to see more attention being given to arboreal ants, and studying their networks will hopefully help us better understand how constraints shape collective behavior. There is a lot to learn on the biological side, of course, but I am eager to see how we can apply what we learn about their transport networks to human-made networks. Perhaps we can identify simple rules that drive “intelligent” collective behavior and apply them to algorithms to create robust, dynamical, and efficient networks.
Soldier poking out of entrance (© Scott Powell). Tree with vine crosscut (© Scott Powell).
In the updated version, the copyright information for three pictures was changed.
Recent Comments